Session 83 - Music Industry
Tracks
Room D1.07 - CCI
Monday, June 24, 2024 |
16:00 - 17:30 |
Speaker
Thomas Paris
Cnrs / Hec Paris
Thomas Serban von Davier
Department Of Computer Science, University Of Oxford
Using Networks to Explore the Invisible Connections in the Music Industry
Extended Abstract
Issue and Arguments
Previous research argues that belonging to a specific network of artists and outlets, such as museums and galleries, is beneficial to an artist's success (Fraiberger et al., 2018). Through network research, the researchers revealed artists with access to certain prestigious museums and galleries increase their chances of success. However, it applies only partially to the music industry. Indeed, while access to legendary venues (i.e., Carnegie Hall or La Scala di Milano) might impact the career of some musicians, access to an “invisible” network of collaborators, producers, composers, and other peers might have a higher impact on success. Previous research on success in the music industry has focused on characteristics of individual songs (Interiano et al., 2018). Research exploring factors of individual artists focused primarily on the personal details of the artists and their early career experiences (Kang et al., 2022). Therefore, the relationship between different performers, producers, and composers and success in the music industry remains unexplored. Presently, only anecdotal evidence has shown the role and impact of invisible producers creating hits for major contemporary celebrities while remaining relatively unknown (see Kornhaber, 2022 for an exposé).
Understanding how an invisible network of artists establishes the music industry is valuable to agencies and researchers and also offers consumers and artists new ways to experience the industry. Exploring and navigating a network of collaborating musicians offers consumers an organic music discovery experience. Researchers argue that if users want to explore music and diversify their listening, they need to “organically” explore and discover music independently (Anderson et al., 2020; Freeman et al., 2023). Failing to engage in active searching and content discovery leads users towards content that serves the platform's goal of generating more advertising hours (Vonderau, 2019). This challenge is underscored by data that suggests approximately 20% of Spotify’s catalog goes unheard by users due to the prioritization of advertisements over music and artists (Palermino, 2014). Therefore, allowing consumers to explore a network of collaborators has potentially opens up the “long tail” of the music industry. Initially described as the Matthew effect (Merton, 1968), the “long tail” states that the discoverability of music diminishes because popular artists dominate the top spots in recommender systems (Celma, 2008). By establishing the network, consumers can look past the big-name artists and better understand the invisible connections that lead to related music, sounds, and other experiences.
For artists, the value of visualizing the network of connections within the music industry reveals the various roles and work that go into the entire industry. In the current age of music streaming, the details regarding credit and attribution have become obscured in favor of recommending and sharing the primary billing artist's name. Due to pushback, Spotify, in particular, has taken steps to enhance the credit and attribution of recommended music throughout the platform (2018). This was followed by pages for listed credited songwriters (Deahl, 2020). However, these improvements have yet to include credits for engineers, studio musicians, and other recording artists who play a crucial role in creating the music hosted on these platforms.
Our work seeks to explore these invisible relationships by analyzing a dataset composed of artists within the pop music domain. Our objective is to describe the relationships between different actors in the music industry and to determine the importance of some nodes (defined as a group of individuals that entertain a certain number of relationships). By aiming to be more proactive in presenting information about credits and artist collaboration relationships, we enable users to explore and engage these connections. Through this network, we can delve into research inquiries such as identifying the nodes which play central roles in the music industry, as well as how artist and label networks shape the musical communities and genres that define our listening experiences today.
Methodology
Using the purpose-built MID - Music Intelligence Database from HEC Montreal, we gather a dataset of song releases alongside the names and IDs of the artists who worked on said releases. This dataset was used to create a network graph comprising artists on the nodes and their collaboration relationships as the edges (Newman, 2010). The more collaborations, the greater weight was given to their connecting edge. Similarly, the size of the nodes was determined by the artist's success defined as number of monthly listeners. With our network established, we ran a variety of analyses on individual nodes and groups of nodes together.
Drawing on established literature that explores the impact of network relationships within the area of art and other creative industries, we selected common approaches to network analysis to understand the roles of artists in the network and their sub-communities. Using eigenvector centrality, we can rank the artists within the network on how connected they are and how connected their connections are within the network (Bonacich, 1987). This ranking enables us to investigate the interplay between network centrality and connectivity and the success metrics favored by large-scale platforms. Taking this analysis a step further than previous musician network research (South, 2017), we can uncover a roadmap to success for emerging artists and expose the hidden connections that drive the music industry. This approach mirrors efforts in the visual art market, where similar network rank and centrality metrics were applied to financial records of art world institutions (Fraiberger et al., 2018). These methods explore questions related to how artists establish themselves in the music industry through their networks and how these collaborations shape the artistic and musical communities in which artists find themselves.
At the time of submission, we have accumulated the database and are working on visualizing and exploring the network graph. Within three weeks, we expect to have a detailed analysis of the various connectivity measurements.
Takeaway and Results
We present three primary takeaways: A purpose-built music database, a usable network graph of a portion of the music industry, and the findings of various mathematical investigations along the nodes and edges of the graph. Additional value of our contribution will come from presenting the results and information to users of large-scale music platforms. By using qualitative consumer research methods (Caru et al., 2014; Creusen et al., 2012), we can explore how expanding their perspective on the music industry will impact their selection and interaction with music. Additionally, by allowing the users to interact with the network graph, we can elicit new reactions and feedback to better inform music information recommendations (see Alvarado and Waern, 2018 on algorithmic experiences). Ultimately, the results will evaluate the network’s relevant metrics and data and present a degree of impact that having the network visualizations will improve a consumer’s experience with online music exploration.
References
Alvarado, O., & Waern, A. (2018). Towards algorithmic experience: Initial efforts for social media contexts. In Proceedings of the 2018 CHI conference on human factors in computing systems (pp. 1-12).
Anderson, A., Maystre, L., Anderson, I., Mehrotra, R., & Lalmas, M. (2020). Algorithmic effects on the diversity of consumption on spotify. In Proceedings of the web conference 2020 (pp. 2155-2165).
Bonacich, P. (1987). Power and centrality: A family of measures. American journal of sociology, 92(5), 1170-1182.
Carù, A., Cova, B., & Pace, S. (2014). Combining qualitative methods in practice: A contextualized account of the evolution of consumer studies. Management Decision, 52(4), 777-793.
Celma, Ò., & Cano, P. (2008). From hits to niches? or how popular artists can bias music recommendation and discovery. In Proceedings of the 2nd KDD Workshop on Large-Scale Recommender Systems and the Netflix Prize Competition (pp. 1-8).
Creusen, M., Hultink, E. J., & Eling, K. (2013). Choice of consumer research methods in the front end of new product development. International Journal of Market Research, 55(1), 81-104.
Deahl, D. (2020). Spotify now lets you search by songwriter with clickable credits. The Verge.
Fraiberger, S. P., Sinatra, R., Resch, M., Riedl, C., & Barabási, A. L. (2018). Quantifying reputation and success in art. Science, 362(6416), 825-829.
Freeman, S., Gibbs, M., & Nansen, B. (2023). Personalised But Impersonal: Listeners' Experiences of Algorithmic Curation on Music Streaming Services. In Proceedings of the 2023 CHI Conference on Human Factors in Computing Systems (pp. 1-14).
Interiano, M., Kazemi, K., Wang, L., Yang, J., Yu, Z., & Komarova, N. L. (2018). Musical trends and predictability of success in contemporary songs in and out of the top charts. Royal Society open science, 5(5), 171274.
Kang, I., Mandulak, M., & Szymanski, B. K. (2022). Analyzing and predicting success of professional musicians. Scientific Reports, 12(1), 21838.
Kornharber, S. (2022). Decoding the most important songwriter of our time. The Atlantic.
Merton, R. K. (1968). The Matthew effect in science: The reward and communication systems of science are considered. Science, 159(3810), 56-63.
N.a. (2018). Spotify now displays songwriter credits. Spotify For Artists.
Newman, M.E.J. (2010). Networks: An Introduction. Oxford University Press.
Palermino, C.L. (2014). Forgotify Plays Spotify’s 4 Million Unheard Songs. Billboard.
South, T. (2018). Network analysis of the Spotify artist collaboration graph. Australian Mathematical Sciences Institute, 1-12.
Vonderau, P. (2019). The Spotify effect: Digital distribution and financial growth. Television & New Media, 20(1), 3-19.
Previous research argues that belonging to a specific network of artists and outlets, such as museums and galleries, is beneficial to an artist's success (Fraiberger et al., 2018). Through network research, the researchers revealed artists with access to certain prestigious museums and galleries increase their chances of success. However, it applies only partially to the music industry. Indeed, while access to legendary venues (i.e., Carnegie Hall or La Scala di Milano) might impact the career of some musicians, access to an “invisible” network of collaborators, producers, composers, and other peers might have a higher impact on success. Previous research on success in the music industry has focused on characteristics of individual songs (Interiano et al., 2018). Research exploring factors of individual artists focused primarily on the personal details of the artists and their early career experiences (Kang et al., 2022). Therefore, the relationship between different performers, producers, and composers and success in the music industry remains unexplored. Presently, only anecdotal evidence has shown the role and impact of invisible producers creating hits for major contemporary celebrities while remaining relatively unknown (see Kornhaber, 2022 for an exposé).
Understanding how an invisible network of artists establishes the music industry is valuable to agencies and researchers and also offers consumers and artists new ways to experience the industry. Exploring and navigating a network of collaborating musicians offers consumers an organic music discovery experience. Researchers argue that if users want to explore music and diversify their listening, they need to “organically” explore and discover music independently (Anderson et al., 2020; Freeman et al., 2023). Failing to engage in active searching and content discovery leads users towards content that serves the platform's goal of generating more advertising hours (Vonderau, 2019). This challenge is underscored by data that suggests approximately 20% of Spotify’s catalog goes unheard by users due to the prioritization of advertisements over music and artists (Palermino, 2014). Therefore, allowing consumers to explore a network of collaborators has potentially opens up the “long tail” of the music industry. Initially described as the Matthew effect (Merton, 1968), the “long tail” states that the discoverability of music diminishes because popular artists dominate the top spots in recommender systems (Celma, 2008). By establishing the network, consumers can look past the big-name artists and better understand the invisible connections that lead to related music, sounds, and other experiences.
For artists, the value of visualizing the network of connections within the music industry reveals the various roles and work that go into the entire industry. In the current age of music streaming, the details regarding credit and attribution have become obscured in favor of recommending and sharing the primary billing artist's name. Due to pushback, Spotify, in particular, has taken steps to enhance the credit and attribution of recommended music throughout the platform (2018). This was followed by pages for listed credited songwriters (Deahl, 2020). However, these improvements have yet to include credits for engineers, studio musicians, and other recording artists who play a crucial role in creating the music hosted on these platforms.
Our work seeks to explore these invisible relationships by analyzing a dataset composed of artists within the pop music domain. Our objective is to describe the relationships between different actors in the music industry and to determine the importance of some nodes (defined as a group of individuals that entertain a certain number of relationships). By aiming to be more proactive in presenting information about credits and artist collaboration relationships, we enable users to explore and engage these connections. Through this network, we can delve into research inquiries such as identifying the nodes which play central roles in the music industry, as well as how artist and label networks shape the musical communities and genres that define our listening experiences today.
Methodology
Using the purpose-built MID - Music Intelligence Database from HEC Montreal, we gather a dataset of song releases alongside the names and IDs of the artists who worked on said releases. This dataset was used to create a network graph comprising artists on the nodes and their collaboration relationships as the edges (Newman, 2010). The more collaborations, the greater weight was given to their connecting edge. Similarly, the size of the nodes was determined by the artist's success defined as number of monthly listeners. With our network established, we ran a variety of analyses on individual nodes and groups of nodes together.
Drawing on established literature that explores the impact of network relationships within the area of art and other creative industries, we selected common approaches to network analysis to understand the roles of artists in the network and their sub-communities. Using eigenvector centrality, we can rank the artists within the network on how connected they are and how connected their connections are within the network (Bonacich, 1987). This ranking enables us to investigate the interplay between network centrality and connectivity and the success metrics favored by large-scale platforms. Taking this analysis a step further than previous musician network research (South, 2017), we can uncover a roadmap to success for emerging artists and expose the hidden connections that drive the music industry. This approach mirrors efforts in the visual art market, where similar network rank and centrality metrics were applied to financial records of art world institutions (Fraiberger et al., 2018). These methods explore questions related to how artists establish themselves in the music industry through their networks and how these collaborations shape the artistic and musical communities in which artists find themselves.
At the time of submission, we have accumulated the database and are working on visualizing and exploring the network graph. Within three weeks, we expect to have a detailed analysis of the various connectivity measurements.
Takeaway and Results
We present three primary takeaways: A purpose-built music database, a usable network graph of a portion of the music industry, and the findings of various mathematical investigations along the nodes and edges of the graph. Additional value of our contribution will come from presenting the results and information to users of large-scale music platforms. By using qualitative consumer research methods (Caru et al., 2014; Creusen et al., 2012), we can explore how expanding their perspective on the music industry will impact their selection and interaction with music. Additionally, by allowing the users to interact with the network graph, we can elicit new reactions and feedback to better inform music information recommendations (see Alvarado and Waern, 2018 on algorithmic experiences). Ultimately, the results will evaluate the network’s relevant metrics and data and present a degree of impact that having the network visualizations will improve a consumer’s experience with online music exploration.
References
Alvarado, O., & Waern, A. (2018). Towards algorithmic experience: Initial efforts for social media contexts. In Proceedings of the 2018 CHI conference on human factors in computing systems (pp. 1-12).
Anderson, A., Maystre, L., Anderson, I., Mehrotra, R., & Lalmas, M. (2020). Algorithmic effects on the diversity of consumption on spotify. In Proceedings of the web conference 2020 (pp. 2155-2165).
Bonacich, P. (1987). Power and centrality: A family of measures. American journal of sociology, 92(5), 1170-1182.
Carù, A., Cova, B., & Pace, S. (2014). Combining qualitative methods in practice: A contextualized account of the evolution of consumer studies. Management Decision, 52(4), 777-793.
Celma, Ò., & Cano, P. (2008). From hits to niches? or how popular artists can bias music recommendation and discovery. In Proceedings of the 2nd KDD Workshop on Large-Scale Recommender Systems and the Netflix Prize Competition (pp. 1-8).
Creusen, M., Hultink, E. J., & Eling, K. (2013). Choice of consumer research methods in the front end of new product development. International Journal of Market Research, 55(1), 81-104.
Deahl, D. (2020). Spotify now lets you search by songwriter with clickable credits. The Verge.
Fraiberger, S. P., Sinatra, R., Resch, M., Riedl, C., & Barabási, A. L. (2018). Quantifying reputation and success in art. Science, 362(6416), 825-829.
Freeman, S., Gibbs, M., & Nansen, B. (2023). Personalised But Impersonal: Listeners' Experiences of Algorithmic Curation on Music Streaming Services. In Proceedings of the 2023 CHI Conference on Human Factors in Computing Systems (pp. 1-14).
Interiano, M., Kazemi, K., Wang, L., Yang, J., Yu, Z., & Komarova, N. L. (2018). Musical trends and predictability of success in contemporary songs in and out of the top charts. Royal Society open science, 5(5), 171274.
Kang, I., Mandulak, M., & Szymanski, B. K. (2022). Analyzing and predicting success of professional musicians. Scientific Reports, 12(1), 21838.
Kornharber, S. (2022). Decoding the most important songwriter of our time. The Atlantic.
Merton, R. K. (1968). The Matthew effect in science: The reward and communication systems of science are considered. Science, 159(3810), 56-63.
N.a. (2018). Spotify now displays songwriter credits. Spotify For Artists.
Newman, M.E.J. (2010). Networks: An Introduction. Oxford University Press.
Palermino, C.L. (2014). Forgotify Plays Spotify’s 4 Million Unheard Songs. Billboard.
South, T. (2018). Network analysis of the Spotify artist collaboration graph. Australian Mathematical Sciences Institute, 1-12.
Vonderau, P. (2019). The Spotify effect: Digital distribution and financial growth. Television & New Media, 20(1), 3-19.
Claudia Chibici-revneanu
UNAM
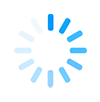